Understanding Data Lifecycle Management
The Basics: What is Data Lifecycle Management?
Data Lifecycle Management (DLM) refers to the processes involved in managing the flow of data throughout its lifecycle, from creation and initial storage to the eventual archival and disposal. The goal of DLM is to optimize the use of data within an organization, ensuring accessibility, reliability, and security while minimizing costs and compliance risks.
Historical Evolution: From Data Storage to Strategic Management
Historically, data management was predominantly about storing information in a safe, retrievable manner. However, as technology evolved and regulatory requirements became more stringent, organizations recognized the need to manage data more strategically. This shift led to the development of comprehensive data lifecycle frameworks that not only handle storage but also focus on how data is created, used, maintained, and eventually retired.
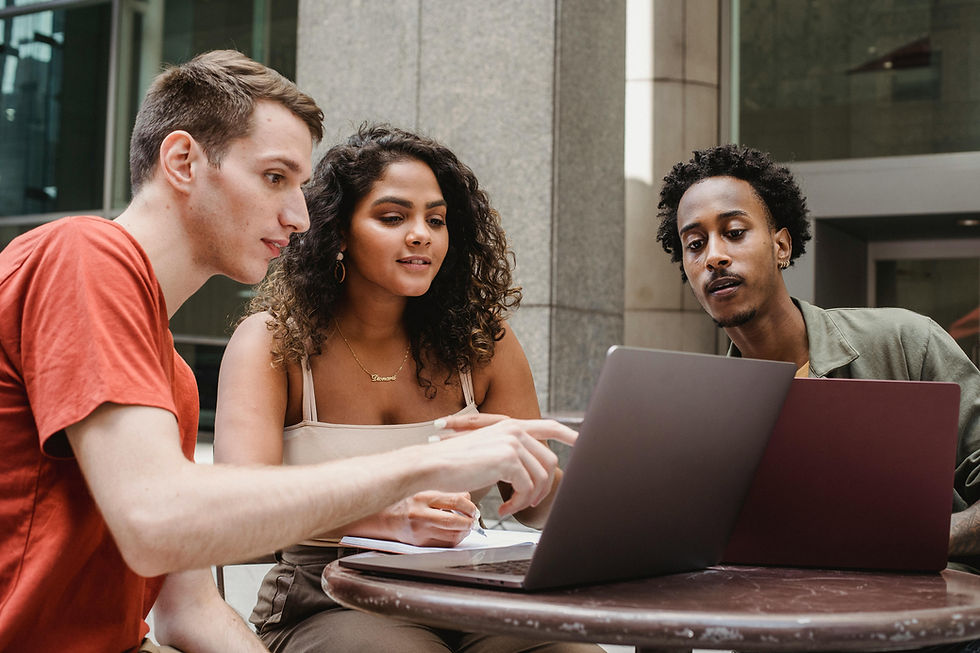
The First Step: Data Creation and Acquisition
Generating Data: Quality Over Quantity
The adage "quality over quantity" is particularly applicable to data creation. High-quality data is accurate, timely, and relevant, reducing the need for costly corrections and ensuring better decision-making. To achieve this, organizations must establish rigorous data governance standards from the outset.
Outsourcing Data Collection: Pros and Cons
Outsourcing data collection can be advantageous, offering access to specialized skills and potentially reducing costs. However, it also comes with risks such as loss of control over data quality and security concerns. Weighing these pros and cons is crucial for determining the best strategy for data acquisition.
Effective Storage Solutions
Scalable Storage Options
As data volumes grow, scalable storage solutions become crucial. These systems allow organizations to increase storage capacity seamlessly without significant disruptions or downtime, ensuring that growing data demands are met efficiently and economically.
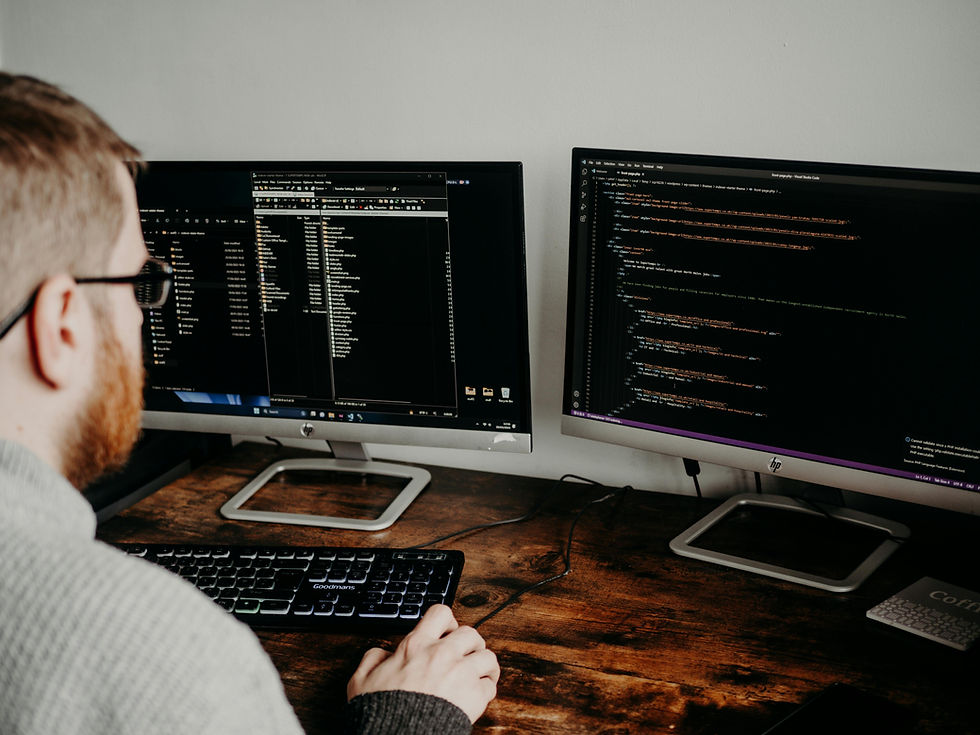
Security Measures in Data Storage
Security is paramount in data storage. Effective measures include encryption, robust access controls, and regular security audits. These practices help protect data against unauthorized access and breaches, which can have devastating reputational and financial impacts.
Cost Implications of Various Storage Technologies
Different storage technologies come with varying cost profiles. Traditional on-premises storage may involve high initial capital outlays, whereas cloud storage offers a pay-as-you-go model that can be more scalable and cost-effective. Balancing these options depends on specific business needs and data sensitivity.
Data Usage: Maximizing Value
Strategic Data Utilization Across Business Functions
Data can significantly enhance business functions when used strategically. From improving customer relationships through detailed analytics to optimizing supply chains, the intelligent use of data can drive innovation and efficiency across all areas of a company.
Ensuring Data Quality During Utilization
Maintaining data quality throughout its use is vital. This involves processes to continuously validate and clean data, ensuring that it remains accurate and useful for decision-making purposes. It is crucial to have robust mechanisms in place to detect and correct any quality issues promptly.
Real-Time Data: Leveraging Instant Insights
Real-time data utilization involves processing data as soon as it is acquired, providing immediate insights that can be used for quick decision-making. This capability is especially important in environments where conditions change rapidly, such as financial markets or emergency response scenarios.
Maintaining Data Accuracy and Quality
Routine Audits for Data Integrity
Regular audits are essential to ensure data integrity. These audits help identify discrepancies and anomalies that could affect data accuracy, providing an opportunity to correct issues before they impact business outcomes.
Tools and Techniques for Data Cleaning
Effective data cleaning tools and techniques include software that can automatically detect and resolve inconsistencies, duplicate data, and incomplete information. Regular use of these tools helps maintain the cleanliness of data, ensuring that it remains functional and reliable for business applications.
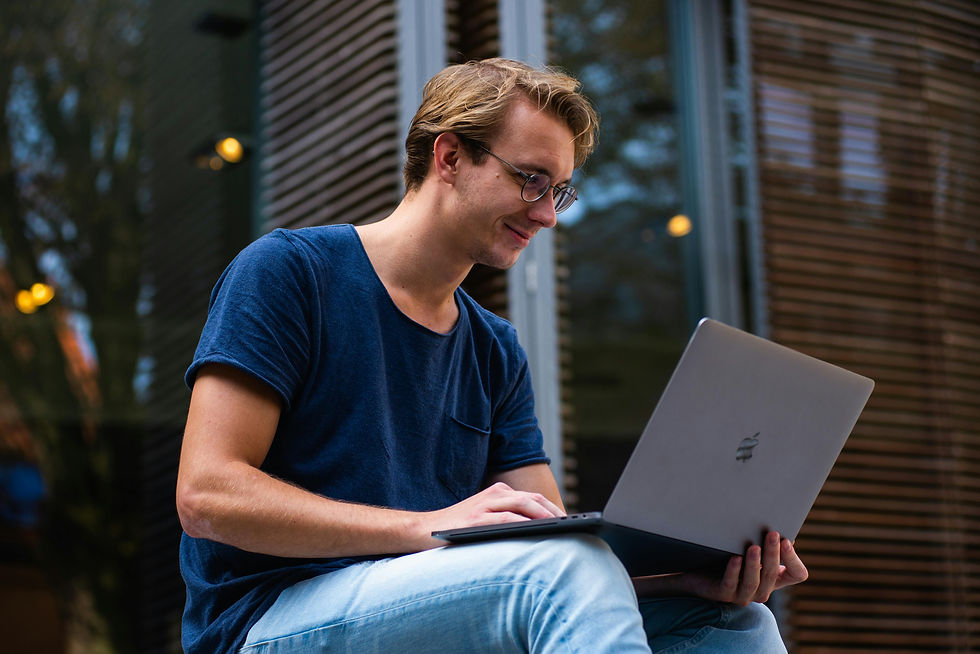
The Role of AI in Data Maintenance
Artificial intelligence (AI) plays a critical role in maintaining data quality. AI algorithms can predict and identify data anomalies faster than manual processes, enabling proactive maintenance and refinement of data sets. This not only enhances data accuracy but also frees up human resources to focus on more strategic tasks.
At ECS LEAD, we integrate AI-driven processes to enhance data quality for our clients. Leveraging advanced AI technologies, we ensure that the data not only remains accurate and up-to-date but also aligns with strategic business goals. By trusting us with your data lifecycle management, you benefit from our expertise in maintaining high data integrity, which is pivotal in today’s data-driven world.
Data Lifecycle Management: Compliance and Risk Management
Adhering to GDPR, CCPA, and Other Regulations
Navigating the complex landscape of data privacy regulations like the General Data Protection Regulation (GDPR) and California Consumer Privacy Act (CCPA) is critical for any organization. Compliance ensures that data handling practices are legally sound and builds trust with consumers who are increasingly concerned about privacy.
Risk Assessment in Data Lifecycle Phases
Risk assessment is integral at each phase of the data lifecycle. By identifying potential vulnerabilities early, from data creation to disposal, organizations can implement appropriate mitigation strategies, reducing the likelihood of data breaches or compliance failures.
Building a Compliance-First Culture
Establishing a compliance-first culture involves training employees to understand and prioritize regulatory requirements. This cultural shift ensures that compliance is considered in every decision related to data management, thereby embedding it into the organization’s DNA.
Archiving and Disposal of Data
Deciding What to Keep and What to Delete
Determining which data to retain and which to dispose of is crucial for managing costs and compliance risks. Data that is no longer necessary should be deleted to avoid clutter and reduce the risk of it being compromised.
Methods for Safe Data Deletion
Proper data deletion methods ensure that information cannot be recovered or misused. Techniques such as data wiping, degaussing, or physical destruction are used depending on the sensitivity of the data and the media on which it is stored.
Long-Term Data Archival Strategies
Long-term data archival strategies involve storing important data in a secure, accessible, and cost-effective manner. Whether using cloud solutions or specialized archival systems, these strategies must protect data integrity and ensure it remains retrievable over time.
Future-Proofing Your Data Management Strategy
Predictive Analytics for Data Lifecycle Optimization
Utilizing predictive analytics can streamline the data lifecycle by forecasting future data trends and needs. This foresight allows organizations to prepare and adapt their strategies to handle changes efficiently.
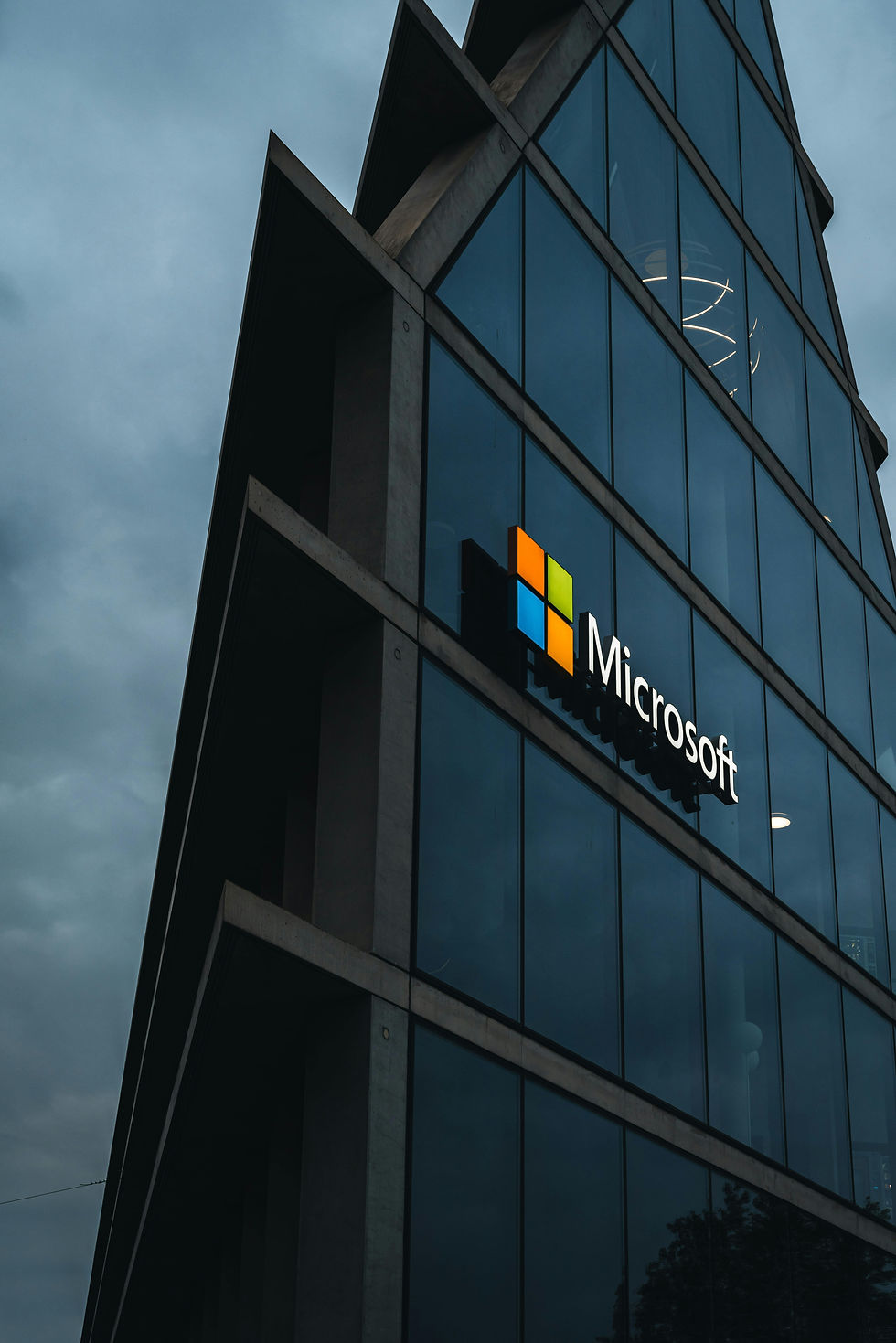
The Impact of Emerging Technologies on Data Strategies
Emerging technologies like blockchain and IoT are transforming data strategies. These technologies offer new ways to secure, process, and integrate data into daily business operations, necessitating continuous updates to data lifecycle management practices.
Best Practices in Data Lifecycle Management
Collaboration Across Departments
Effective data lifecycle management requires collaboration across various departments. This unified approach ensures that data policies are universally understood and implemented, enhancing overall efficiency and data quality.
Continuous Learning and Adaptation
As the digital landscape evolves, so must data management strategies. Organizations must commit to continuous learning and adaptation to embrace new technologies, processes, and regulatory changes.
Benchmarking Against Industry Standards
Benchmarking against industry standards is crucial for maintaining a competitive edge. It helps identify gaps in an organization’s data strategy and drives improvements by adopting best practices.
The Bigger Picture: Strategic Business Impacts
Case Studies: Success Stories and Failures
Learning from both successes and failures in data management offers valuable insights. Case studies illustrate the practical impacts of effective data lifecycle management or the consequences of oversight.
Data Management as a Business Driver
When managed well, data can significantly drive business success. It informs strategic decisions, enhances customer relationships, and optimizes operations, proving to be a pivotal asset in the competitive market.
Beyond the Basics: Advanced Data Lifecycle Considerations
The Growing Role of Cloud Computing
Cloud computing has become integral to modern data strategies. It offers scalable, flexible, and cost-effective solutions for storing and managing vast amounts of data while supporting advanced analytics capabilities.
Balancing Innovation with Practicality in Data Management
While innovation is essential, it must be balanced with practicality. Organizations should adopt new technologies and practices that are not only forward-thinking but also applicable and beneficial in their specific operational contexts.